基于ResNet网络模型的手术切口常见特征的识别
Deep Learning-Based Identification of Common Complication Features of Surgical Incisions
-
摘要:目的 近年来由于加速康复外科及日间手术在外科领域的发展,使得患者平均住院日缩短,术后手术切口需居家康复,为及时发现伤口存在的问题,预防或减轻患者出院后的焦虑,本研究利用深度学习的方法对手术切口常见并发症的特征进行分类,期望实现以患者为主导的手术切口常见并发症的早期识别。方法 收集2021年6月−2022年3月某三甲医院手术后患者的切口图像1224张,根据并发症特征进行分类整理,并将其按8∶1∶1的比例分为训练集、验证集和测试集,使用4种卷积神经网络分别进行模型的训练与测试。结果 通过多种卷积神经网络的训练,并在基于300张手术切口图像测试集的基础上进行模型性能的测试,4种ResNet分类网络模型SE-ResNet101、ResNet50、ResNet101、SE-ResNet50的手术切口分类平均准确率分别为0.941、0.903、0.896、0.918,精确率分别为0.939、0.898、0.868、0.903,召回率分别为0.930、0.880、0.850、0.894,其中以SE-Resnet101网络模型切口特征分类平均准确率最高,达到0.941。结论 将深度学习和手术切口图像相结合的方式,能通过手术切口图像对手术切口的问题特征进行有效识别,最终有望实现患者智能终端手术切口自检。Abstract:Objective In recent years, due to the development of accelerated recovery after surgery and day surgery in the field of surgery, the average length-of-stay of patients has been shortened and patients stay at home for post-surgical recovery and healing of the surgical incisions. In order to identify, in a timely manner, the problems that may appear at the incision site and help patients prevent or reduce the anxiety they may experience after discharge, we used deep learning method in this study to classify the features of common complications of surgical incisions, hoping to realize patient-directed early identification of complications common to surgical incisions.Methods A total of 1224 postoperative photographs of patients' surgical incisions were taken and collected at a tertiary-care hospital between June 2021 and March 2022. The photographs were collated and categorized according to different features of complications of the surgical incisions. Then, the photographs were divided into training, validation, and test sets at the ratio of 8∶1∶1 and 4 types of convolutional neural networks were applied in the training and testing of the models.Results Through the training of multiple convolutional neural networks and the testing of the model performance on the basis of a test set of 300 surgical incision images, the average accuracy of the four ResNet classification network models, SE-ResNet101, ResNet50, ResNet101, and SE-ResNet50, for surgical incision classification was 0.941, 0.903, 0.896, and 0.918, respectively, the precision was 0.939, 0.898, 0.868, and 0.903, respectively, and the recall rate was 0.930, 0.880, 0.850, and 0.894, respectively, with the SE-Resnet101 network model showing the highest average accuracy of 0.941 for incision feature classification.Conclusion Through the combined use of deep learning technology and images of surgical incisions, problematic features of surgical incisions can be effectively identified by examining surgical incision images. It is expected that patients will eventually be able to perform self-examination of surgical incisions on smart terminals.
© 2023 《koko体育app
学报(医学版)》编辑部 版权所有
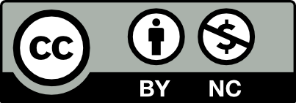
开放获取🎉 本文遵循知识共享署名—非商业性使用4.0国际许可协议(CC BY-NC 4.0),允许第三方对本刊发表的论文自由共享(即在任何媒介以任何形式复制、发行原文)、演绎(即修改、转换或以原文为基础进行创作),必须给出适当的署名,提供指向本文许可协议的链接,同时标明是否对原文作了修改;不得将本文用于商业目的。CC BY-NC 4.0许可协议详情请访问